МЕТОДЫ МАШИННОГО ОБУЧЕНИЯ В ЦИФРОВОМ СЕЛЬСКОМ ХОЗЯЙСТВЕ: АЛГОРИТМЫ И КЕЙСЫ
Аннотация
Обеспечение продовольственной безопасности является важной задачей многих стран. В условиях роста населения Земли вопросы повышения эффективности сельского хозяйства становятся наиболее актуальными. Фермеры ищут новые способы повышения урожайности, а правительства разных стран разрабатывают новые программы поддержки сельского хозяйства. Это способствует более активному внедрению цифровых технологий в сельское хозяйство, помогая фермерам более эффективно принимать решения, увеличивать урожайность и заботиться об экологии. Центральное место здесь занимает сбор и анализ данных. В области земледелия данные могут собираться из разных источников и содержат в себе полезные закономерности, выявляющие потенциальные проблемы или возможности. Чтобы извлечь пользу из данных, они должны быть проанализированы с помощью алгоритмов машинного обучения. Такие методы точного земледелия позволяют следить за отдельными частями поля, оптимизировать расход воды и химикатов, а также оперативно выявлять проблемы.
Цель: обзор алгоритмов машинного обучения, применяемых для анализа данных в сельском хозяйстве.
Методология проведения работы: обзор релевантной литературы, опрос фермеров.
Результаты: выявлены релевантные алгоритмы машинного обучения для анализа данных в сельском хозяйстве на различных уровнях: анализ почвы (оценка состояния почвы, классификация почвы, прогнозироваие плодородности почвы), прогноз погоды (имитация смены климата, прогноз температуры и осадков) и анализ состояния растительности (идентификация сорняков, классификация растительности, выявление болезни растений, прогнозирование урожайности).
Область применения результатов: сельское хозяйство, растениеводство.
Ключевые слова
Полный текст:
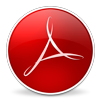
Литература
Al-Hiary H., Bani-Ahmad S., Reyalat M., Braik M. and ALRahamneh Z. “Fast and accurate detection and classification of plant diseases.” Machine learning 14, no. 5 (2011): 31–38.
Anderson-Cook, Christine M., Alley M.M., Roygard J. K. F., Khosla R., Noble R.B. and Doolittle J.A. “Differentiating soil types using electromagnetic conductivity and crop yield maps.” Soil Science Society of America Journal 66, no. 5 (2002): 1562–1570.
Bannayan, Mohammad, and Gerrit Hoogenboom. “Weather analogue: a tool for real-time prediction of daily weather data realizations based on a modified k-nearest neighbor approach.” Environmental Modelling & Software 23, no. 6 (2008): 703–713.
Bhargavi P., and Jyothi S. “Applying naive bayes data mining technique for classification of agricultural land soils.” International journal of computer science and network security9, no. 8 (2009): 117–122.
Camargo A., and Smith J.S. “An image-processing based algorithm to automatically identify plant disease visual symptoms.” Biosystems engineering 102, no. 1 (2009): 9–21.
Douaik, Ahmed, Marc Van Meirvenne, and Tibor Tóth. “Statistical methods for evaluating soil salinity spatial and temporal variability.” Soil Science Society of America Journal71, no. 5 (2007): 1629–1635.
Fernandes, Jeferson Lobato, Jansle Vieira Rocha, and Rubens Augusto Camargo Lamparelli. “Sugarcane yield estimates using time series analysis of spot vegetation images.” Scientia Agricola 68, no. 2 (2011): 139–146.
Fraley, Chris, Adrian Raftery, Tilmann Gneiting, McLean Sloughter, and Veronica Berrocal. “Probabilistic Weather Forecasting in R.” R Journal 3, no. 1 (2011): 55–63.
Gallo, Kevin, Lei Ji, Brad Reed, Jeffery Eidenshink, and John Dwyer. “Multi-platform comparisons of MODIS and AVHRR normalized difference vegetation index data.” Remote Sensing of Environment 99, no. 3 (2005): 221–231.
Geerken, Roland A. “An algorithm to classify and monitor seasonal variations in vegetation phenologies and their inter-annual change.” ISPRS Journal of Photogrammetry and Remote Sensing 64, no. 4 (2009): 422–431.
Gholap Jay. “Performance tuning of J48 Algorithm for prediction of soil fertility.” arXiv preprint arXiv:1208.3943(2012).
Ingsrisawang, Lily, Supawadee Ingsriswang, Saisuda Somchit, Prasert Aungsuratana, and Warawut Khantiyanan. “Machine learning techniques for short-term rain forecasting system in the northeastern part of Thailand.” In Proceedings of World Academy of Science, Engineering and Technology, vol. 31 (2008): 248–253.
Johari A., Habibagahi G. and Ghahramani A. “Prediction of soil–water characteristic curve using genetic programming.” Journal of Geotechnical and Geoenvironmental Engineering132, no. 5 (2006): 661–665.
Johnson, Michael D., William W. Hsieh, Alex J. Cannon, Andrew Davidson, and Frédéric Bédard. “Crop yield forecasting on the Canadian Prairies by remotely sensed vegetation indices and machine learning methods.” Agricultural and forest meteorology 218 (2016): 74–84.
Laliberte, Andrea S., Mark A. Goforth, Caitriana M. Steele, and Albert Rango. “Multispectral remote sensing from unmanned aircraft: Image processing workflows and applications for rangeland environments.” Remote Sensing 3, no. 11 (2011): 2529–2551.
Mar, Khaing Win, and Thinn Thu Naing. “Optimum neural network architecture for precipitation prediction of Myanmar.” World Academy of Science, Engineering and Technology 48 (2008): 130–134.
Mkhabela M.S., Paul Bullock S. Raj S. Wang, and Y. Yang. “Crop yield forecasting on the Canadian Prairies using MODIS NDVI data.” Agricultural and Forest Meteorology 151, no. 3 (2011): 385–393.
Moustris, Kostas P., Ioanna K. Larissi, Panagiotis T. Nastos, and Athanasios G. Paliatsos. “Precipitation forecast using artificial neural networks in specific regions of Greece.” Water resources management 25, no. 8 (2011): 1979–1993.
Nemes, Attila, R.T. Roberts, Walter J. Rawls, Ya.A. Pachepsky, and M. Th Van Genuchten. “Software to estimate− 33 and− 1500 kPa soil water retention using the non-parametric k-nearest neighbor technique.” Environmental Modelling & Software 23, no. 2 (2008): 254–255.
Ortiz-García E.G., Salcedo-Sanz S., and Casanova-Mateo C. “Accurate precipitation prediction with support vector classifiers: A study including novel predictive variables and observational data.” Atmospheric research 139 (2014): 128–136.
Radhika, Y., and M. Shashi. “Atmospheric temperature prediction using support vector machines.” International journal of computer theory and engineering 1, no. 1 (2009): 55–58.
Ramesh, V., and K. Ramar. “Classification of agricultural land soils: a data mining approach.” Agricultural Journal 6, no. 3 (2011): 82–86.
Sharif, Mohammed, and Donald H. Burn. “Simulating climate change scenarios using an improved K-nearest neighbor model.” Journal of hydrology 325, no. 1–4 (2006): 179–196.
Vibhute, Anup, and S. K. Bodhe. “Applications of image processing in agriculture: a survey.” International Journal of Computer Applications 52, no. 2 (2012): 34–40.
Zhou, Zhi-Hua. Ensemble methods: foundations and algorithms. CRC press, 2012.
DOI: https://doi.org/10.12731/2227-930X-2018-1-11-26
Ссылки
- На текущий момент ссылки отсутствуют.
(c) 2018 Aleksandr Vasilyevich Koshkarov

Это произведение доступно по лицензии Creative Commons «Attribution-NonCommercial-NoDerivatives» («Атрибуция — Некоммерческое использование — Без производных произведений») 4.0 Всемирная.
Контент доступен под лицензией Creative Commons Attribution-NonCommercial-NoDerivs 4.0.
ISSN 2328-1391 (print), ISSN 2227-930X (online)